Brightness Augmentation Implementation to Evaluate Perfor-mance Classification of Face Masked Base on CNN Model
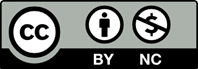
The use of Deep Learning methods with CNN models is starting to be applied such as in Facial Expression Recognition. However, with the pandemic situation in recent years, there are still some people who wear masks for work purposes or because they are sick so that their faces are not fully visible. This can affect social interactions, especially in the mouth area which is very informative. Therefore, this research aims to provide a better understanding of masked facial expression recognition with the application of CNN models, namely with VGG16 and MobileNet architectures. In addition, this research will also explore the use of data augmentation methods, such as geometric augmentation and brightness augmentation, to see their effect on the classification accuracy of masked facial expressions. The results show that the use of VGG16 architecture with cross-validation method (VGG16-FLCV) provides better performance than MobileNet-FLCV architecture in recognizing and classifying masked facial expressions. The application of data augmentation methods, such as geometric augmentation and brightness augmentation, has helped to improve the performance of CNN models. Experimental results show that on the VGG16-FLCV architecture, the brightness range (1.00, 1.25) provides the best accuracy with a training accuracy of 81.73% and a validation accuracy of 70.71%. In addition, this study found that the optimal use of brightness range on the VGG16-FLCV architecture is in the darkness category with ranges (0.25, 0.50), (0.50, 0.75), and (0.75, 1.00), as well as in the brightness category with ranges (1.00, 1.25). This study found that the MobileNet-FLCV architecture with a brightness range of (0.25, 0.50), (0.50, 0.75), (0.75, 1.00), (1.00, 0.25), and (1.25, 1.50) can be used as an alternative brightness range that can still be applied without experiencing a significant decrease in accuracy.